Land ho! Now what?
Without a map or the notion of a GPS, ancient explorers ventured into unfamiliar territory searching for a place to settle. Cliffs, steep mountains and impassable rivers thwarted their efforts as time ticked on and supplies dwindled.
Drug hunters face a similar challenge. When scientists discover a new disease-related biological mechanism, researchers looking for ways to interrupt that mechanism have a continent’s worth of possible chemical options to navigate – without a signpost in sight.
To help, Novartis researchers have developed a guidance system for drug discovery that employs digital technologies such as machine learning and automation to help steer them toward optimal compounds to bring forward as potential medicines. The technology, which is already in use at the company, could not only accelerate the early drug discovery process but also help teams find better leads on drugs than they could in the past. The system is part of a larger effort to apply data and digital tools at Novartis that help make drug discovery and development more efficient and intelligent.
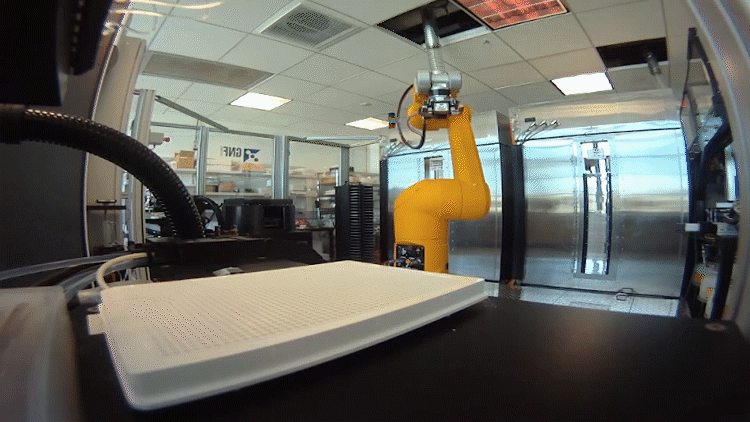
The novel technology – internally called MicroCycle – makes it possible for researchers to iteratively test and evaluate 100 unique chemicals in the time it used to take to evaluate a handful of options. By exploring and learning from many more compounds, they have a better chance of finding a promising lead on a new medicine.
“We were trying to optimize drugs for patients, but we would only have time and resources to try a few options”, says Jonathan Grob, a Novartis chemist involved in creating the system. “What we really wanted to do was compress that cycle time – and make each cycle smarter – so we could have a better chance at finding something good”.
Make, test, learn
The process begins with a hit, an experiment revealing that a given compound perturbs a disease process. The next step is to make many more compounds that might improve on it.
We try to see the bigger picture from the start.
Alex Marziale
The challenge is that there is no obvious way to know which compounds to make. The possibilities are practically endless.
To help, the Novartis team developed a machine learning system that suggests recipes. The team trained the system by feeding it information about the many compounds already in use at Novartis, the same way computer scientists have trained machine learning systems to learn to make new food recipes from existing ones.
If you’ve ever seen a food recipe generated by machine learning, you’ll know that what comes out is sometimes…unexpected. Brownies made with a cup of horseradish, anyone?

But that is the beauty of the system. The team’s goal is to explore the chemical space widely and not shy away from places they would normally avoid. There may be something to learn from adding that horseradish to the mix.
"We’ve had suggestions where the chemists have actually said, ‘Ew, that looks horrible,’” says Cara Brocklehurst, a Novartis chemist who helped envision and now oversees the project. “But guess what? We’ll make it anyway and learn from it”.
In fact, in one instance, the team felt skeptical about a suggested recipe. They didn’t expect much from it. But testing revealed that the compound was potent at altering the disease biology and it also had nice drug properties.
“With this GPS approach, you have to detach yourself from expectations about single molecules and instead focus on how knowledge can guide the direction of the project”, says Brocklehurst. “This forced us out of our comfort zone and into working in a completely different way”.
Seeing the big picture
Learning about multiple aspects of a compound – not only its ability to perturb the disease process but also its drug properties, such as solubility or cell permeability – is a critical part of the process. In the past, these properties were often taken one at a time, the same way ancient explorers with no maps might have approached the quest for a settlement place, choosing to walk an easy path, finding a water source, then exploring nearby for food.
A drug discovery GPS system enables a more holistic approach. The team can think about and improve upon all of the properties of the drug at once.
“We try to see the bigger picture from the start. Your GPS isn’t only showing a two-dimensional landscape. It’s also showing hills and valleys plus travel time and more”, says Alex Marziale, a Novartis chemist also involved in creating the system.
In the very beginning, the path forward isn’t any clearer than it might have been without the guidance, but the view of the landscape clears with each iteration.
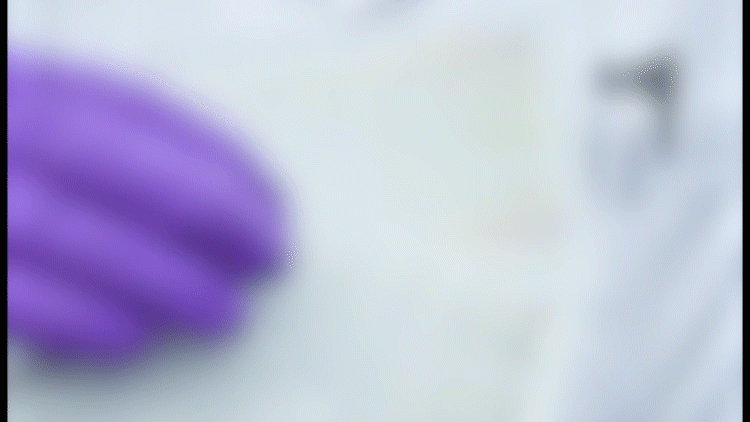
“It’s like the clouds will disappear. You get a list of compounds that you make and that helps you fill in the space”, says Brocklehurst. “Then the next time around, you get a clearer picture“.
Miniaturized science
The system relies on automated testing of compounds in many different scenarios in parallel. To accomplish this, the team – an interdisciplinary group of chemists, data scientists, biologists and analysts – had to shrink the chemistry lab.
In the past, drug hunters might make 20 to 50 milligrams of a chemical to be tested. That was fine when they made a handful of compounds. Now they make hundreds of recipes in tiny batches a fraction of that size.
They need so little because the plates used to test the compounds in biological scenarios are densely populated with miniature wells. Each well performs a unique experiment. The entire system, from plating to reading the results, is automated using robots.
The amount of data emerging from these high-throughput tests would be overwhelming for a human. Imagine tasting 50 batches of brownie recipes to see which is best.
“In the end, it becomes difficult to tell the difference”, says Grob.
So the team feeds the results back into the machine learning system. It uses the data to learn how each compound performed, then virtually creates another list of compounds to try. The team repeats the process until they stop learning new things.
In the best case, they’ve found a lead on a compound that could become a drug candidate. In the worst case, they’ve encountered cliffs, steep mountains and impassable rivers at every turn.
“We may get to a dead end, but we get there fast and we learn a lot”, says Grob.
Novartis researchers have developed a guidance system to make #drugdiscovery more efficient and intelligent.